Is facial recognition helping or hurting law enforcement?
While the majority of people in the United States approve of the police using facial recognition technology, experts warn that some may be relying on it too heavily, and that the level of human involvement still required cannot be underestimated. However, the solution may simply lie in better governance and increased transparency.
In 2018, a man was accused of stealing a pair of socks from a department store in New York. The man was first identified by a facial recognition algorithm fed with a security camera image. Officer Jones then sent the match to a security guard working at the store. Is this the guy? she asked. The guard confirmed it. The suspect was arrested. However, he could not have stolen the socks. At the time of the theft, he was at the hospital where his son was being born.
A non-profit organisation called Thorn, for example, uses facial recognition to scan online ads to spot underage victims of sex trafficking, while in counter-terrorism, facial recognition software frequently reduces the time needed to generate vital early leads.
The risk of wrongful arrest is one of the main concerns surrounding the use of facial recognition by law enforcement. Yet on the other hand, the technology has helped law enforcement to speed up investigations, accurately identify criminals and prevent crimes on countless occasions.
So is facial recognition a friend or a foe to law enforcement? As this question remains unanswered, experts analyse all the shortcomings and solutions.
What happens when facial recognition finds a match?
Law enforcement agencies use facial recognition searches to identify criminal suspects or missing persons. A common example is a thief caught on CCTV. The CCTV-image is compared against a database of images of people whose identities are known, such as a collection of mugshots or driving license photographs, and the perpetrator can be identified if there is a match.
While the technological performance of the algorithm is therefore of great importance, this is only one part of a long workflow that still involves many human decisions.
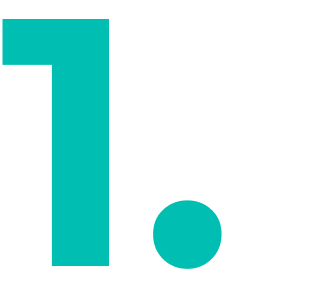
Picking the photo.
Facial recognition searches usually start with a clue, such as an image from a security camera. The choice of photo is quite important. If the image is pixelated or blurred, or the person’s face is turned away from the camera, the search results will be less reliable.
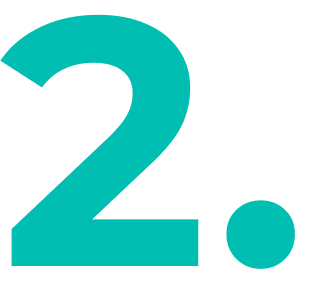
Automated search.
The photo is run through a system that compares it against a large database of images, such as a collection of mugshots. The facial recognition algorithms perform these searches automatically, and the accuracy of each algorithm varies depending on the mathematical model used and the dataset on which it was trained. Newer algorithms tend to be more accurate.
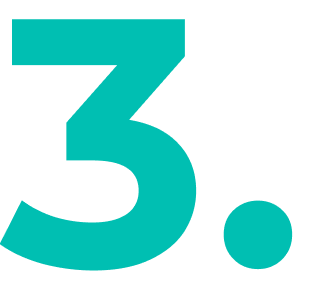
Photo editing.
If the search does not produce valid results, a human officer can edit the original image with the aim of making it more consistent with the photos stored in the database. For example, they can enlarge the photo or use blur and sharpening tools where the photo lacks detail.
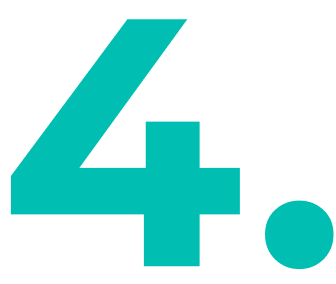
Human review.
The facial recognition search returns a “candidate list” of possible matches, ranked according to a confidence score the algorithm has generated. The candidate list should always be reviewed by a human officer, who may decide to add further details to the list, such as the confidence score, previous criminal arrests and other information related to the case.
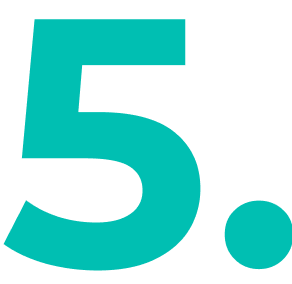
Investigative follow-up.
Most police departments instruct their officers to treat a facial recognition match as an investigative lead rather than a 100%-positive identification. Investigators might show a selection of potential matches to an eyewitness for identification, or they can cross-reference the results with other clues that emerge during the investigation.
What can go wrong in a facial recognition search?
Because facial recognition is a multi-step process, many variables come into play: how new and accurate is the algorithm being used by the police department? What input photo is fed into the algorithm? How is the list of suspects presented to witnesses for their confirmation or identification?
The public tends to focus solely on how accurate the software is.
However, an algorithm’s reliability depends on many factors. These include the way the neural network was constructed, the choices made by the developer, the dataset used to train the algorithm and how the training has been carried out. The result also depends on the quality of the input photo. Some algorithms also perform better or worse depending on the race, gender and age of the person being searched.
One of the biggest concerns at present is that algorithms could disproportionately harm black people, as the technology often has higher error rates for people with dark skin.
The Innovatrics facial recognition algorithm has scored top marks in accuracy and speed in the FRVT evaluation.
Read more on how the accuracy of facial recognition technology has improved over time.
Is the issue of bias still relevant?
To evaluate the role of bias in facial recognition algorithms, we need relevant data coming from trustworthy sources. One such source is the Facial Recognition Vendor Test (FRVT) developed by the National Institute of Standards and Technology (NIST).
FRVT is one of the world’s most respected evaluators of facial recognition algorithms. It evaluates the accuracy of algorithms submitted by vendors around the world in terms of both verification and identification, and challenges the software with demanding tests that involve using different demographic groups or poor-quality images.
Every month, the FRVT releases a report from its Ongoing series, which assesses the performance of facial recognition algorithms across different demographic groups, such as race and gender.
Based on the most recent evaluation, each of the top 150 algorithms are over 99% accurate across black male, white male, black female and white female demographics. Of the top 20 algorithms, accuracy of the highest performing demographic versus the lowest varies by just 0.1%, from 99.8% to 99.7%.
Unexpectedly, white male was the lowest performing of the four demographic groups in the top 20 algorithms.
Not only have NIST evaluations shown that the accuracy of algorithms has improved dramatically in recent years, but they also provide the public and law enforcement agencies with a ranking of the algorithms that perform best in certain areas.
“Each of the top 150 algorithms are over 99% accurate across black male, white male, black female and white female demographics. Unexpectedly, white male is currently the lowest performing of the four demographic groups in the top 20 algorithms.”
How reliable is human identification?
A recent report by the Georgetown Law Center on Privacy & Technology lists all the shortcomings of the use of facial recognition in law enforcement. The authors claim that both the agencies and the public underestimate the significant degree of human judgement involved.
Before and after the input photo is fed to the algorithm, human operators have to select, edit and review the image, all of which can have a significant impact on the reliability of the algorithm’s results.
The leads generated by the technology then require further human involvement, whether from the officers or from witnesses who may be required to make a positive identification.
Buy how reliable is this aspect of human identification? Research has shown that an untrained eye – such as that of a witness or even a police officer – can have error rates varying from around 10 to 60% (page 22-23). However, a 2015 study showed that trained facial forensic examiners – personnel with many years’ experience of comparing face images for law enforcement and government agencies – have significantly lower error rates.
The way forward, according to some experts, is thus to combine algorithms with conscious human input that is controlled by robust policies and transparent workflows.
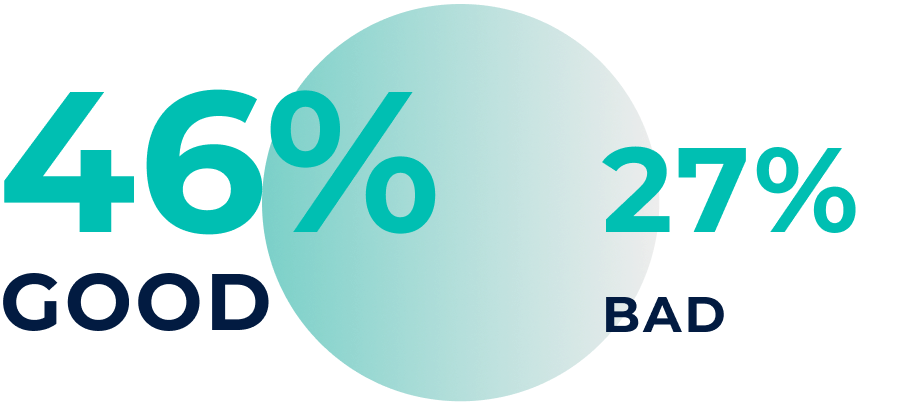
A recent survey in the US found that the public considers the use of facial recognition by police as good for society (46% of respondents) rather than bad (27%).
Total bans or more transparent policies?
Based on the Georgetown Law Center on Privacy & Technology’s report, information about how police forces use facial recognition in criminal investigations remains scarce. Meaning that the public still lacks a clear picture of how and when facial recognition technology is used.
The good news is that in the United States, standard bodies are working to establish minimum photo-quality standards, user guidelines, training programs and other systems to limit mistakes and improve reliability.
“The solution is to Increase the transparency on how the technology is used in criminal investigations and educate communities to its value and risks.”
However, at the same time, cities such as San Francisco, Oakland and Boston have banned the use of facial recognition technology by public agencies in order to avoid any of its potential pitfalls. It’s clear technology alone cannot be the only source of identifying criminals, but is an all-or-nothing approach really the right answer?
According to many observers, there is another option: increase the transparency on how the technology is used in criminal investigations, and educate communities to its value and risks. That means building better governance for the use of facial recognition systems in law enforcement, to ensure that society as a whole can benefit.
AUTHOR: Giovanni Blandino
ILLUSTRATION: Helga Pavelková
Sources
- Facial Recognition Technology: Responsible Use Principles and the Legislative Landscape
- This is best practice for using facial recognition in law enforcement
- The Hidden Role of Facial Recognition Tech in Many Arrests
- A FORENSIC WITHOUT THE SCIENCE
- Perceptual expertise in forensic facial image comparison
- Can police use facial recognition as probable cause?
- A Forensic Without the Science: Face Recognition in U.S. Criminal Investigations