How the accuracy of facial recognition technology has improved over time
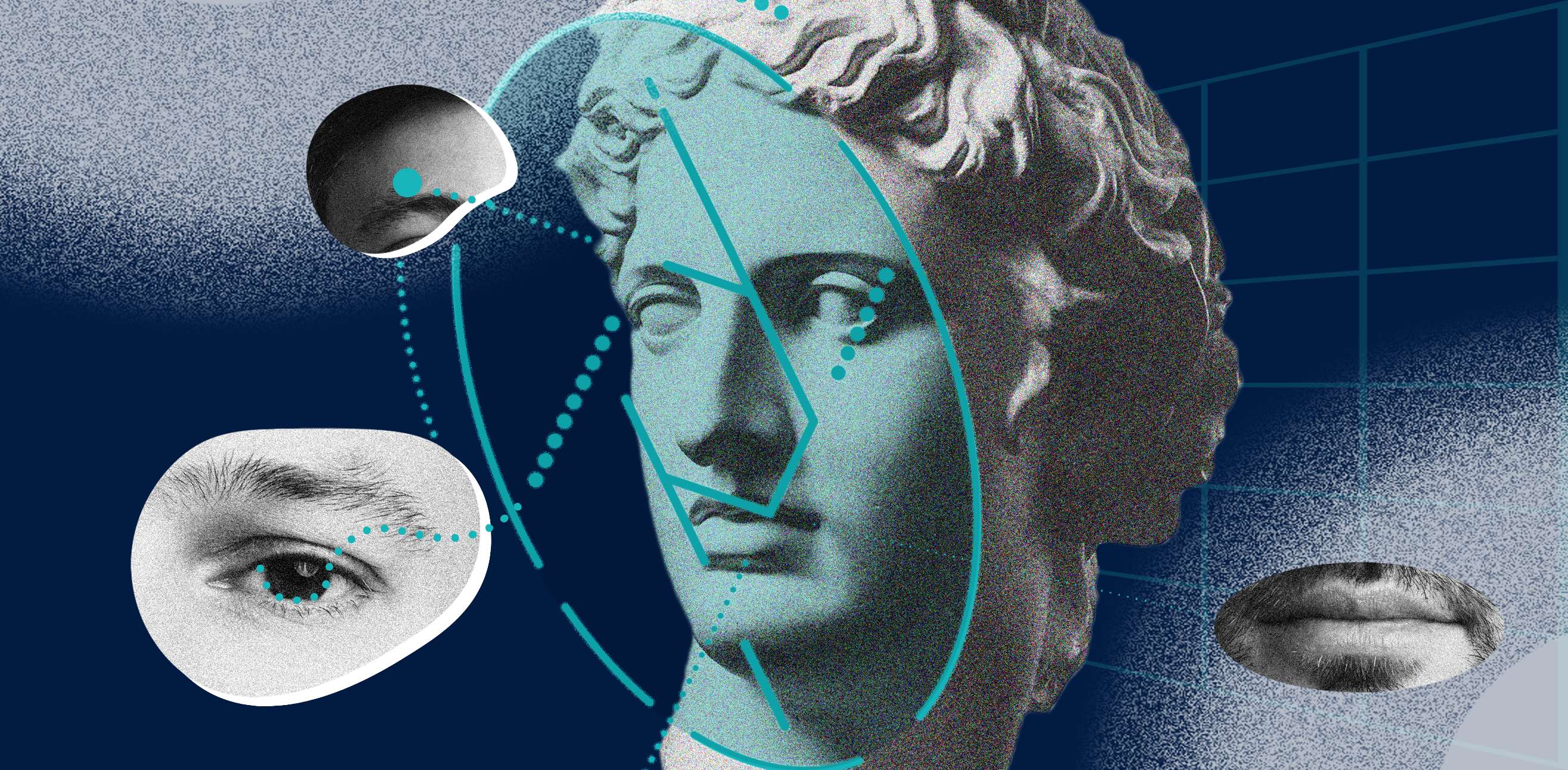
On our way towards a paperless future, Innovatrics has developed seven facial recognition algorithms in the past two years so as to help speed up processes involving facial recognition software. No biometric system is error-free, but year by year the accuracy gets more and more exact. Since 2017, Innovatrics has improved its facial recognition accuracy four times in case of low-quality pictures and 33 times in the case of high-quality ones.
Dive into this explanatory article and see how face-recognition technology has improved over time, how the accuracy of face recognition gets measured and what Innovatrics’ role has been in the overall development.
How to measure the development of facial-recognition algorithms on a global scale
The Innovatrics algorithm has scored top marks in accuracy and speed. These are crucial for large-scale applications such as document verification, criminal investigation and border control. Read more about the November 2020 results here.
To check the progress, the Face Recognition Vendor Test (FRVT) was invented as a benchmark evaluation method of facial-recognition algorithms for verification and identification. Such a test measures the performance of algorithms developed in both commercial and academic communities worldwide to evaluate their competitive advantage. The result is a list of submitted vendors ranked from the lowest to the highest performance.
To execute FRVT correctly, we have to measure two kinds of rates, both dependable on each other.
The first is the false reject rate (FRR, also called false non-match rate or FNMR): the instance of a security system failing to verify or identify an authorised person, hence misreading the data and causing a false rejection. It measures the number of ‘no found matches’ with regards to identical faces.
To measure FRR, we also have to measure the likelihood of a biometric security system incorrectly accepting an access attempt by an unauthorised user. That is called the false accept rate (FAR, also called false match rate or FMR), meaning the number of incorrectly found matches.
How algorithms have been improving
There are several ways to improve the accuracy of facial recognition. The first is “education” – the better the dataset that the neural network is trained on, the better are the results. To help the machine learn better, the datasets have to be labelled correctly and checked for mistakes. The other avenue is to rely on improving computing power. Neural networks can be more precise and their outputs can be tested repeatedly to find and repair their blind spots.
The accuracy of FRVT has to be measured via an FRR (false reject rate) and FAR (false accept rate) ratio; the number of times a camera incorrectly detects a false match (there’s a match found, even if the face is not in the database) to the number of times a camera fails to find a correct match (the camera does not recognise a person’s identity, even though they are already in the database).
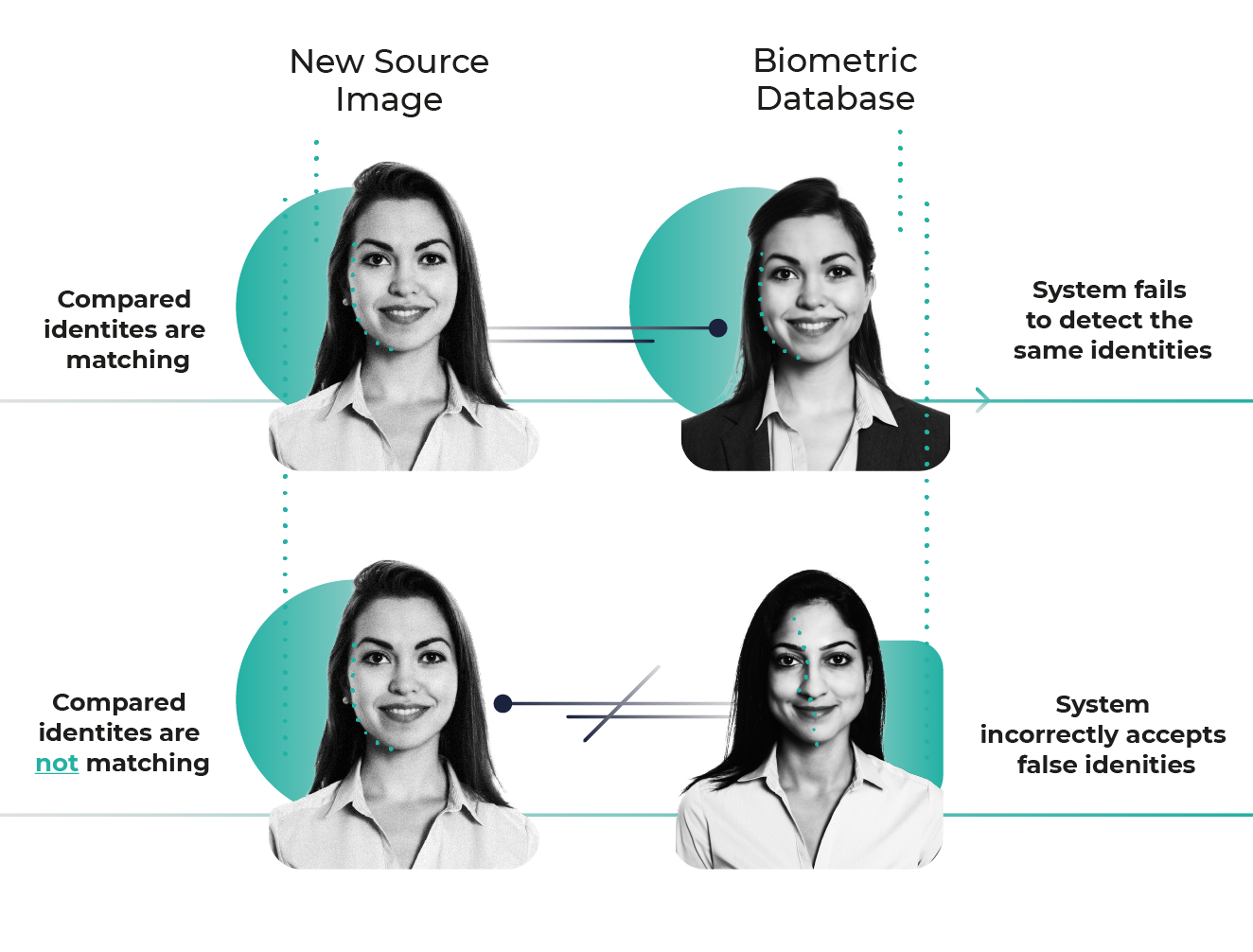
Axis x represents the year of the new algorithm was measured. Each vertical line represents the newest algorithm developed at the time.
Axis y stands for the accuracy ratio of FRR (false reject rate) and FAR (false accept rate) ratio.
The accuracy of Face Recognition
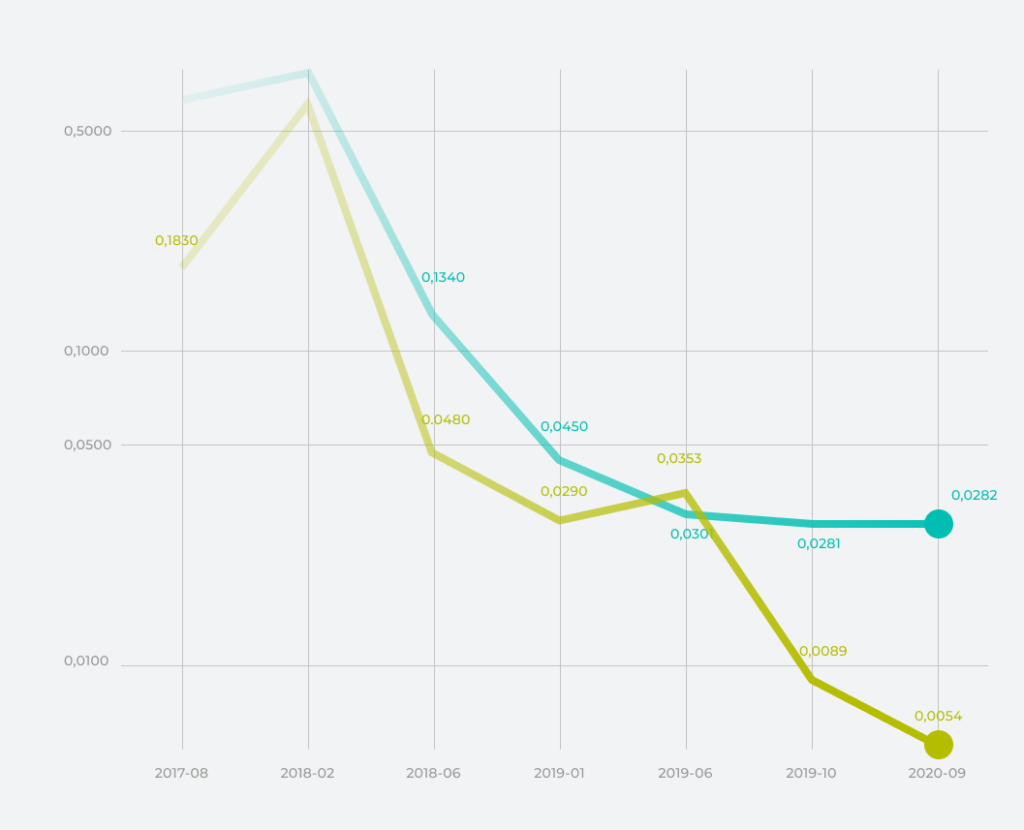
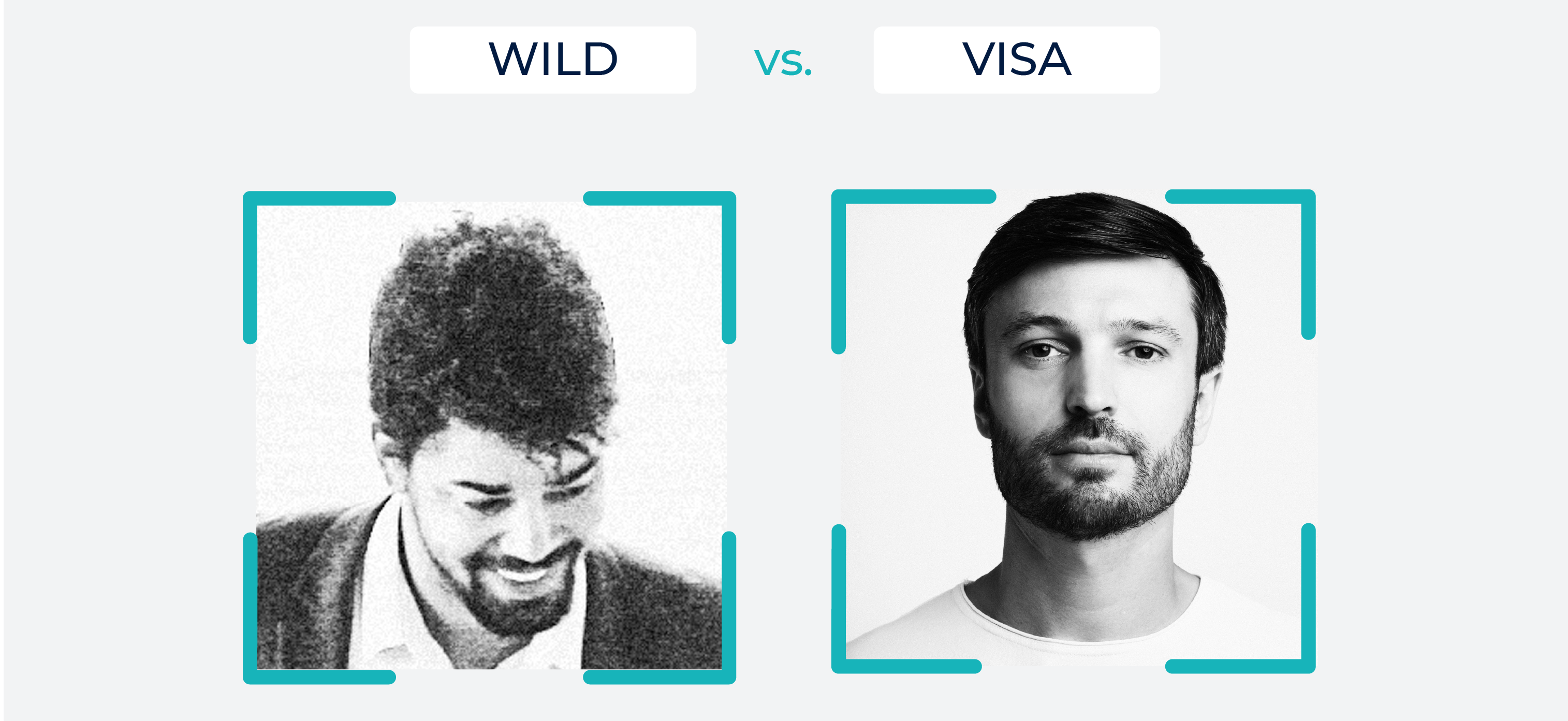
Recently, the ability to recognize faces with face masks came to the fore. Read more about how the algorithms are able to tackle this new phenomenon here.
How the quality of the pictures affects the identification
Taking Wild photography as a source, the algorithms for facial recognition are 4.6 times more accurate today than they were two years ago.
On the quality spectrum, Wild pictures are those of a low-quality nature, taken during the least suitable conditions for face recognition – often captured by street cameras from various angles, with poor light, a face partially covered by hair or a mask, profile-facing images, and such. Wild pictures are much less accurate than Visa pictures, but when it comes to Wild-pictures accuracy recognition, Innovatrics has scored the highest marks in the world.
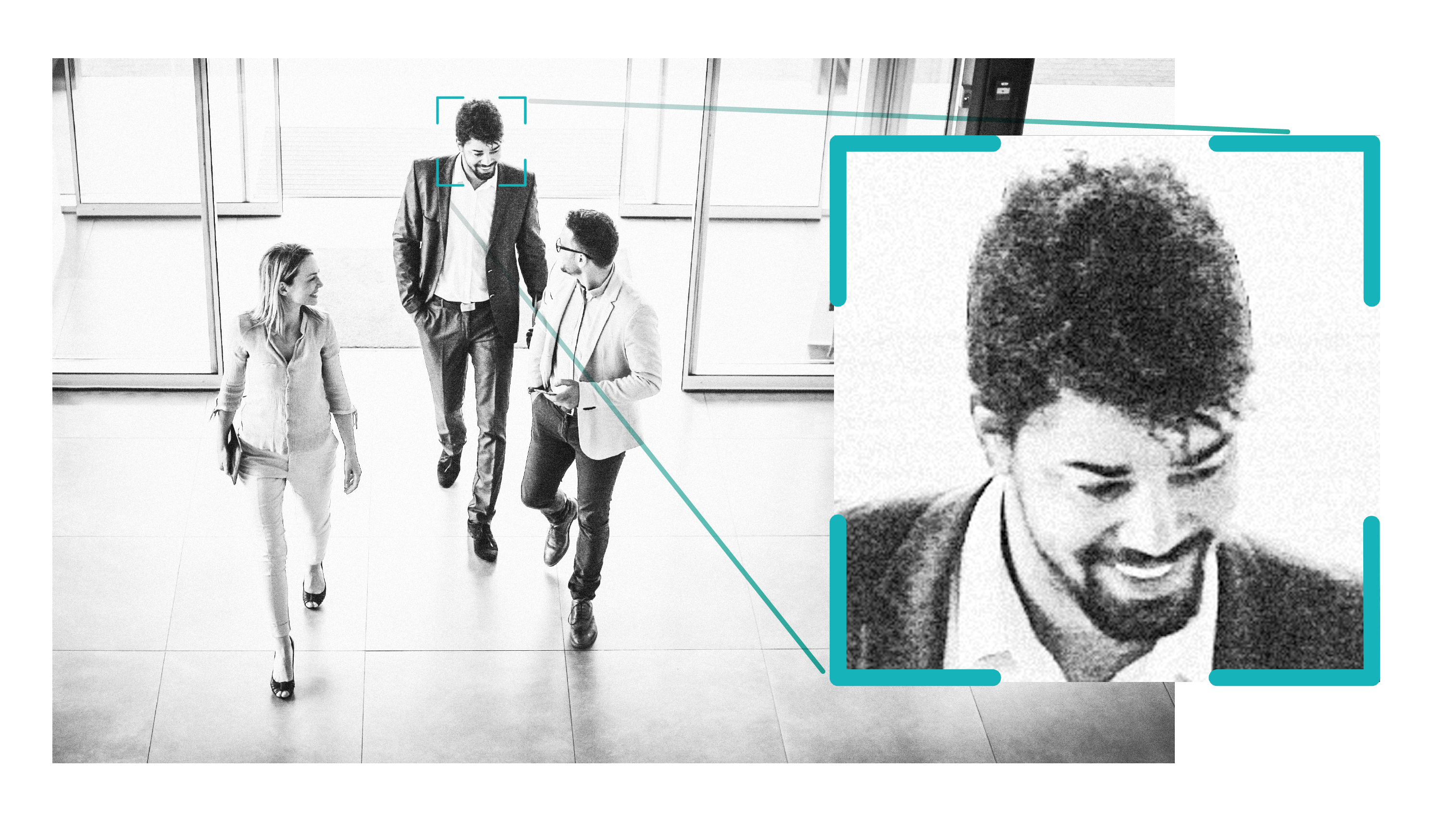
Looking back at the graph, Wild pictures are represented by the green line, which indicates the number of times the system did not recognise a person who passed by the camera, despite their details already being held on file. We can observe the error rate notably dropping since 2017.
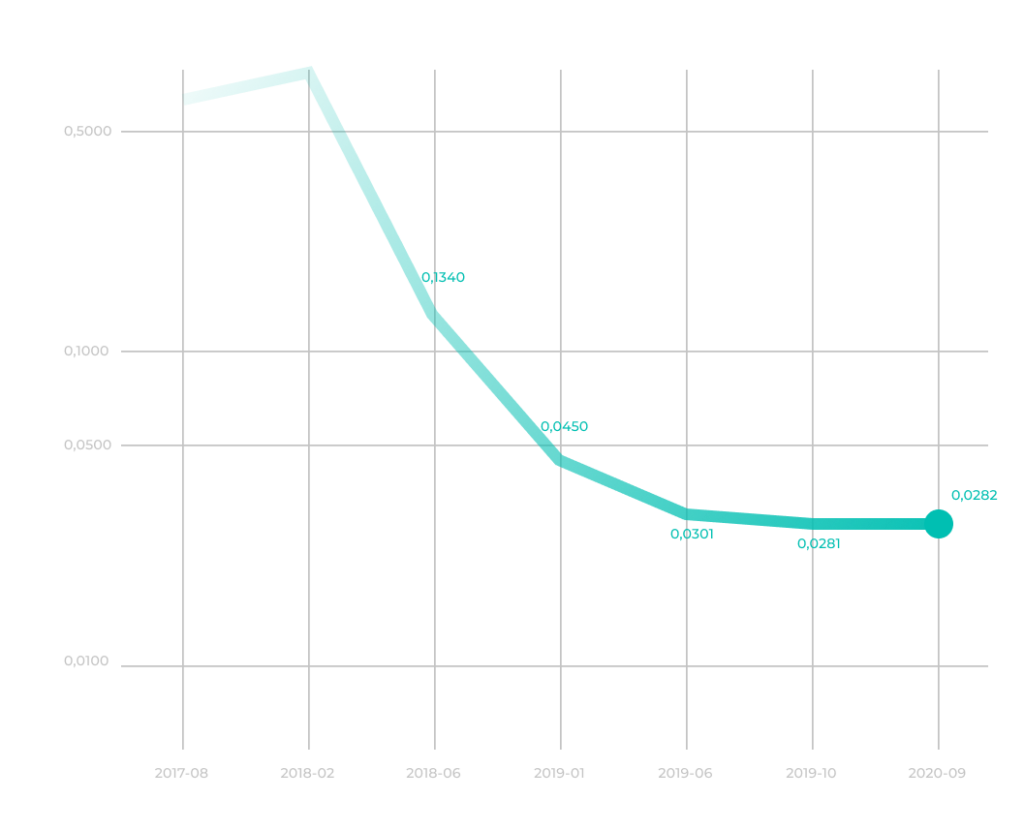
Taking the value (0.028) from September 2020 and comparing it to the value (0.134) from June 2018, we see the algorithms are 4 times more accurate today than they were two years ago. The most recent results show the system did not recognise 0.28 cases out of 10,000 on average, which is 4.6 times more accurate than two years ago. In 2018, it was 1.3 cases out of 10,000.
An example of where face recognition from wild pictures can be used
There’s a camera in front of the building where Peter F. works. The camera is connected to the self-opening door. Given that the camera is well-positioned and with a radius wide enough to capture the walk-ins, it is able to recognise people whose details are already held on file and establish who they are, just by their walking past the camera. As an employee, Peter F. needn’t look directly at the camera nor take his mask off. The match can often be identified correctly and the door opened. In a few cases, however, such as what happened to Peter F. last time, the camera fails to recognise a person. In this instance, Peter F. had to place himself more visibly in front of the camera and take his mask off for a short time, to allow the camera to record his biometric data.
No biometric system is error-free. The question is about how many cases per 10,000 attempts a camera does and does not recognise an employee, as well as how many incorrectly verified and identified people are let into the building by the machine confusing an unregistered person with someone already stored in the database.
Innovatrics has consistently been ranked among the most accurate and fastest algorithms in the world owing to its R&D team, who are committed to the continuous improvement of image processing, neural networks and machine learning. Read more about the results on performance in all categories in this article.
The accuracy with Visa-picture identification has improved by 33 times within just two years.
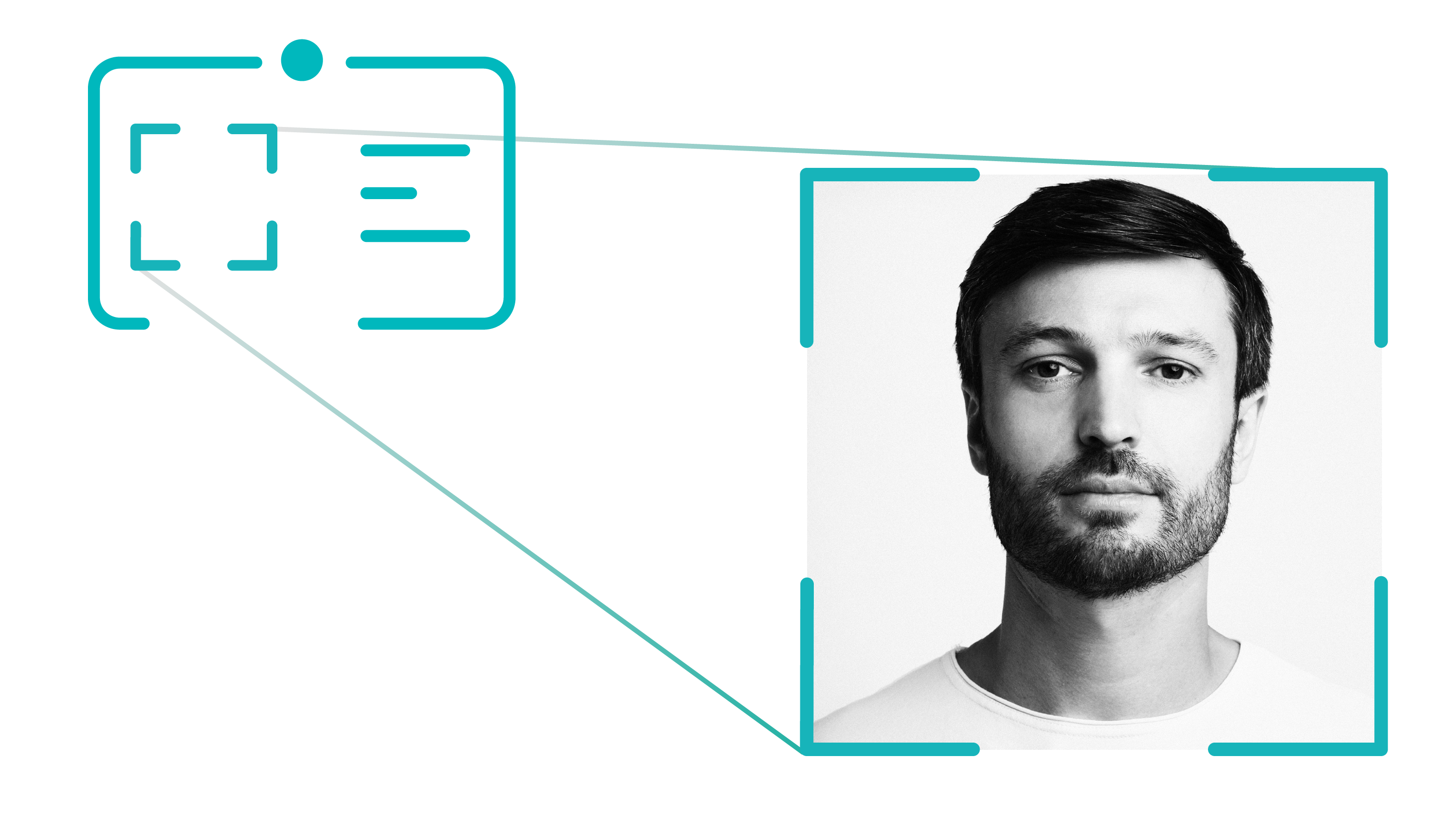
As opposed to Wild pictures, Visa pictures are those of the highest quality, meeting the standard of regular Visa photography requirements, such as the picture having to be taken from the front profile solely, with direct light, open eyes, closed mouth, light background and so. There are international requirements on how a Visa picture should look.
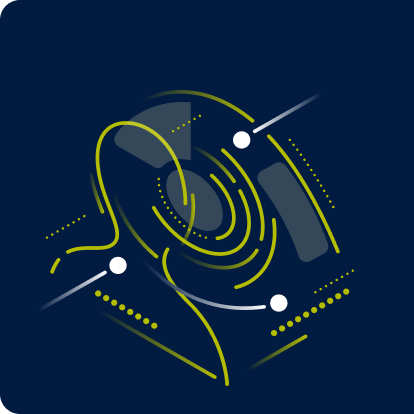
Automated Biometric Identification System Tailored to Your Needs
Innovatrics ABIS is a biometric identity management system supporting fingerprint, iris and facial recognition. Fast and accurate performance is achieved with minimal hardware requirements.
ViewIn the graph, the yellow line indicates Visa pictures. As the line drops on the axis x, the number of times the system did not recognise a person based on the source of the Visa picture decreases as it approaches the current year and date.
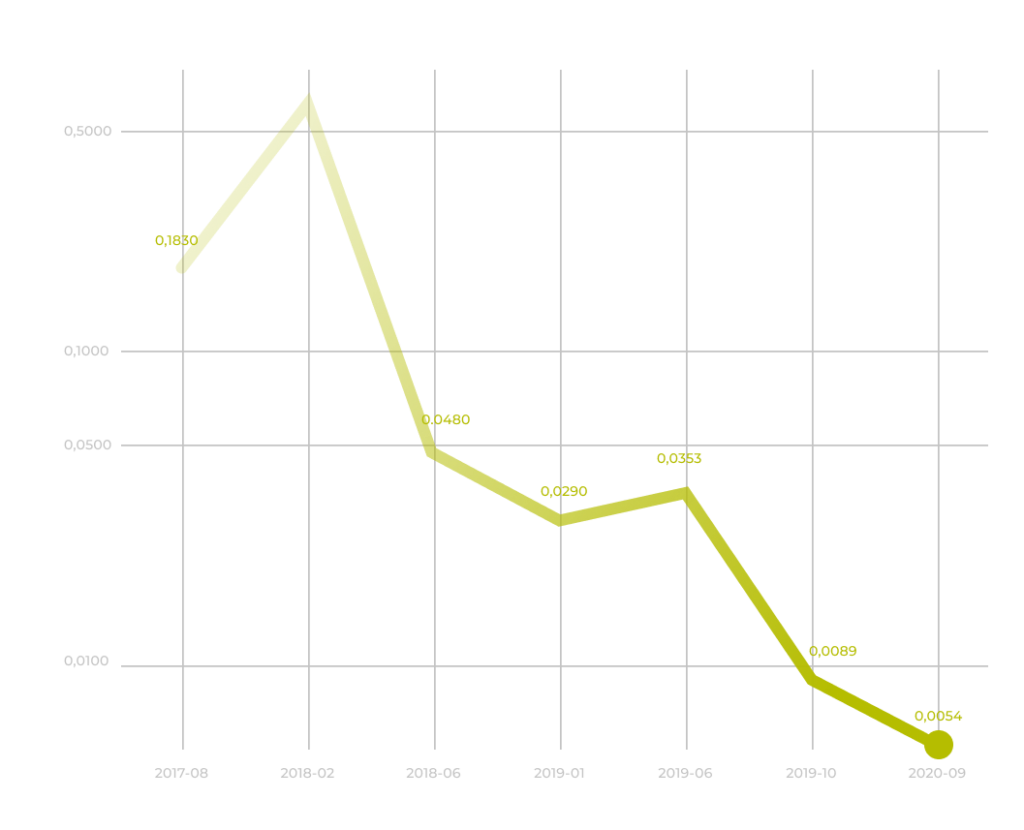
Observing the yellow line in 2018, on average, 0.18 cases out of 1 million failed to be correctly detected. Two years later in 2020, the numbers have greatly improved to an average of only 0.0054 cases out of 1 million.
The accuracy of facial recognition with Visa-picture identification has grown by 33 times within just two years. It is worth mentioning that in this case, the accuracy is easier to reach thanks to the quality of the pictures. On the other hand, each mistake is to be considered much more seriously compared to Wild pictures.
An example of where face recognition from visa pictures can be used
A police officer, Frank S., needs to identify and verify a certain person stored in a police database. He looks for a high-quality Visa picture, as the person concerned had made a new passport with biometric data included, and thus, his picture is filed in the biometric database as well. In such circumstances, the chances are far too slim for a system to incorrectly detect a person. Frank S. swiftly recognises the person and can continue to focus on the next, bigger challenge.
AUTHOR: Barbara Rusnáková
PHOTOS: Shutterstock
GRAPH: Innovatrics