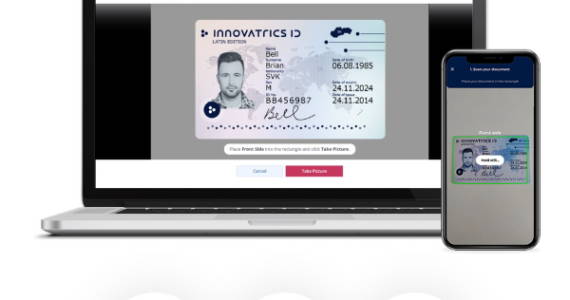
New DOT Web SDK Enables In-Browser Document & Face Auto-Capture
Innovatrics has released DOT Web SDK, a new component of Digital Onboarding Toolkit (DOT), which enables in-browser ID ...
Read moreThe higher quality of images means that our passive liveness algorithm is able to increase its certainty that a given image is of a genuine person – or a fake.
When trying to improve on our passive liveness algorithm – that had already passed iBeta level 2 tests – our R&D team has observed that the edge cases all share the same feature: they are all low quality images. Edge cases are significant for training the neural network as they are on the boundary where an algorithm is not quite sure whether it’s seeing a genuine person, an impostor, or no face at all (some people try to fool the algorithm with doll faces and such).
“Filtering out low quality images allows us to increase the accuracy of the algorithm without compromising customer convenience,” Marian Beszedes, the Head of R&D at Innovatrics, explained when presenting the findings at the conference of European Association for Biometrics.
The approach was based on one of the research papers that spurred a round of testing resulting in a new approach. Just by making a preliminary quality filter when taking a picture, the algorithm is automatically more accurate. The original paper was focused on facial recognition and was looking for gender and ethnicity biases. Our approach shows that the same method can be applied to passive liveness as well.
Passive liveness is a part of the Digital Onboarding Toolkit used by financial institutions and other companies to provide reliable, accurate identification when acquiring new customers remotely. With it, a customer can also get instant feedback on how to improve the picture quality, e.g., by finding a better-lit place or moving away from the backlight. Moreover, an auto-capture feature takes out the guesswork from taking a selfie: the system takes it when conditions are optimal.
The research has also shown that high quality pictures with extra high sharpness are also all spoofs. “The extra sharpness level comes from the pictures containing moire effect, i.e., it’s a photo of another screen showing a photograph,” Marian adds. Adding a simple sharpness detector can therefore also filter out spoofs, further increasing the algorithm’s already high accuracy. “Thanks to quality analysis, we can now focus on high quality spoofs and inaccurately classified genuine selfies,” Marian says.
Passive liveness check has been developed in-house with high accuracy, low hardware requirements and customer convenience in mind. You can also read an in-depth overview of our research here.
Interested in Digital Onboarding Toolkit? Try our demo or contact us to learn more.