Seeing past the marketing fluff: How to identify trustworthy biometric providers
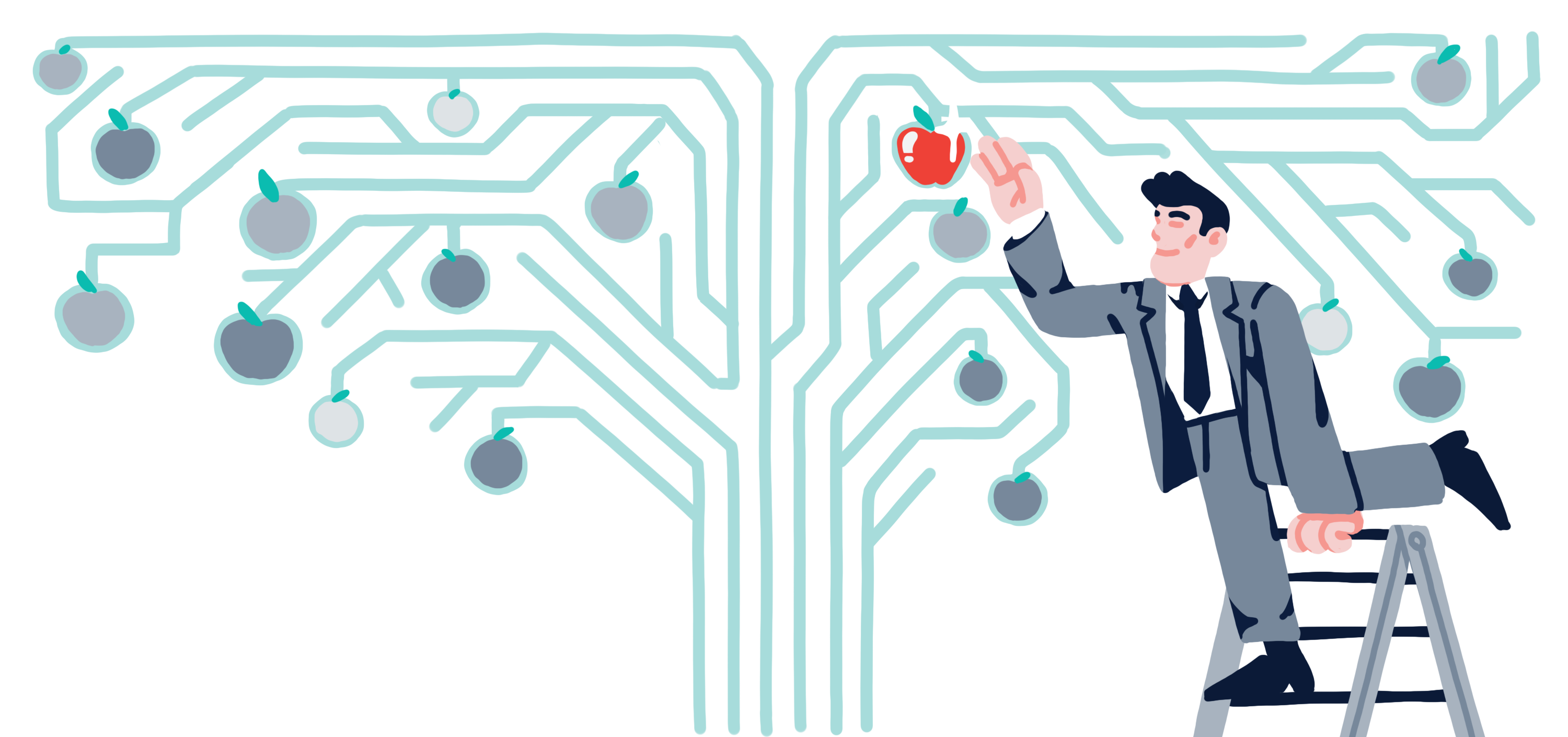
Contributors
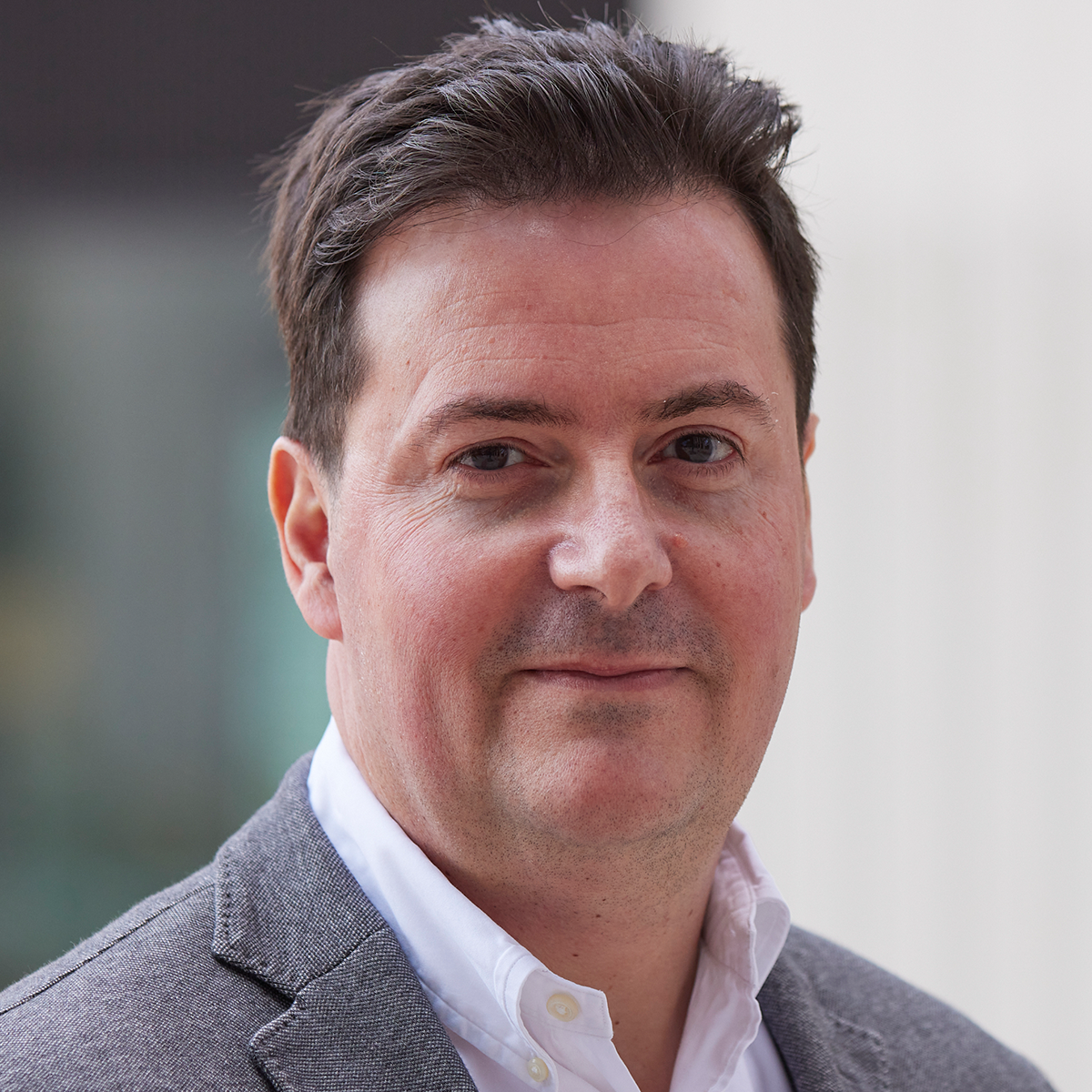
Miguel Traquina
CTO of iProov
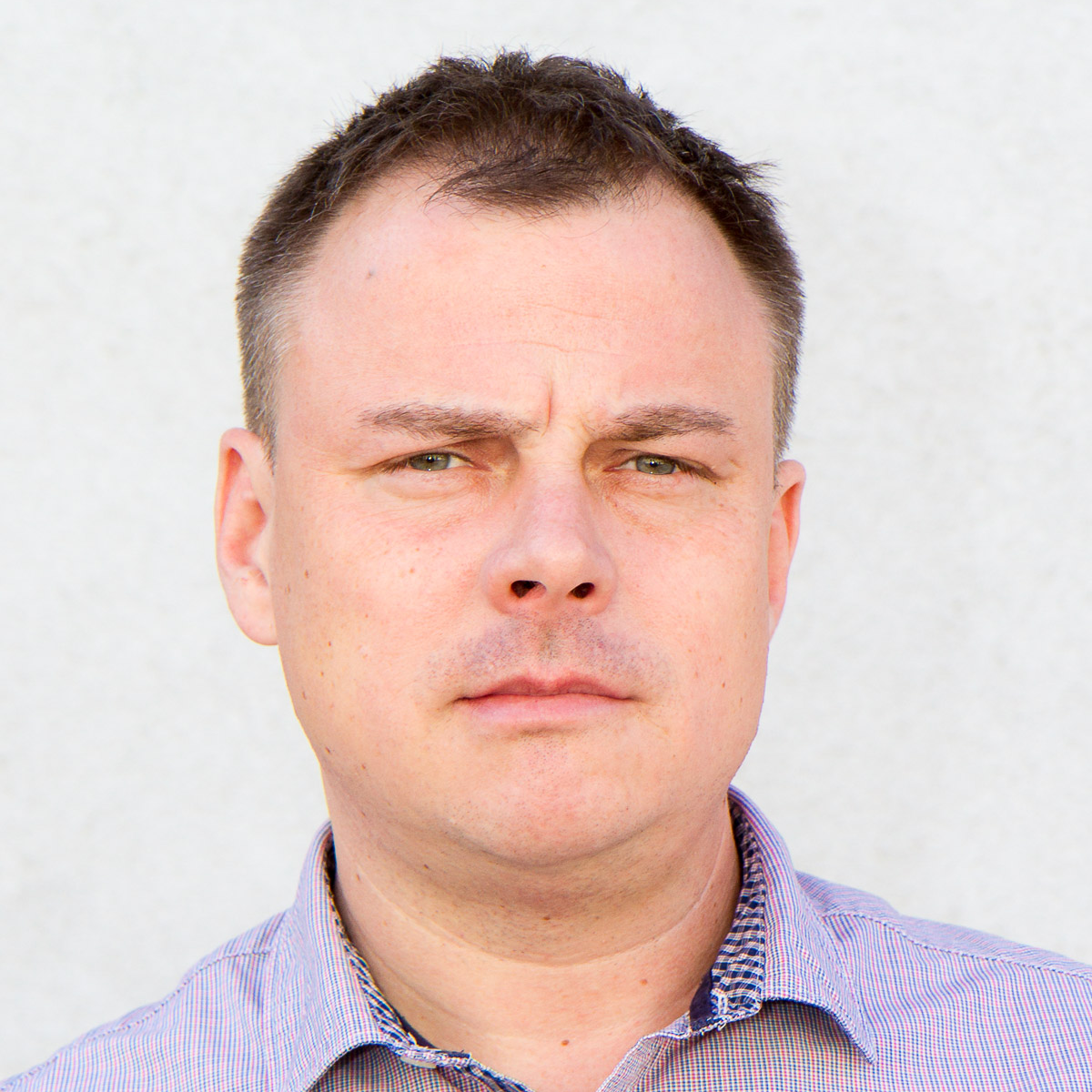
Peter Martis
Head of Global Sales at Innovatrics
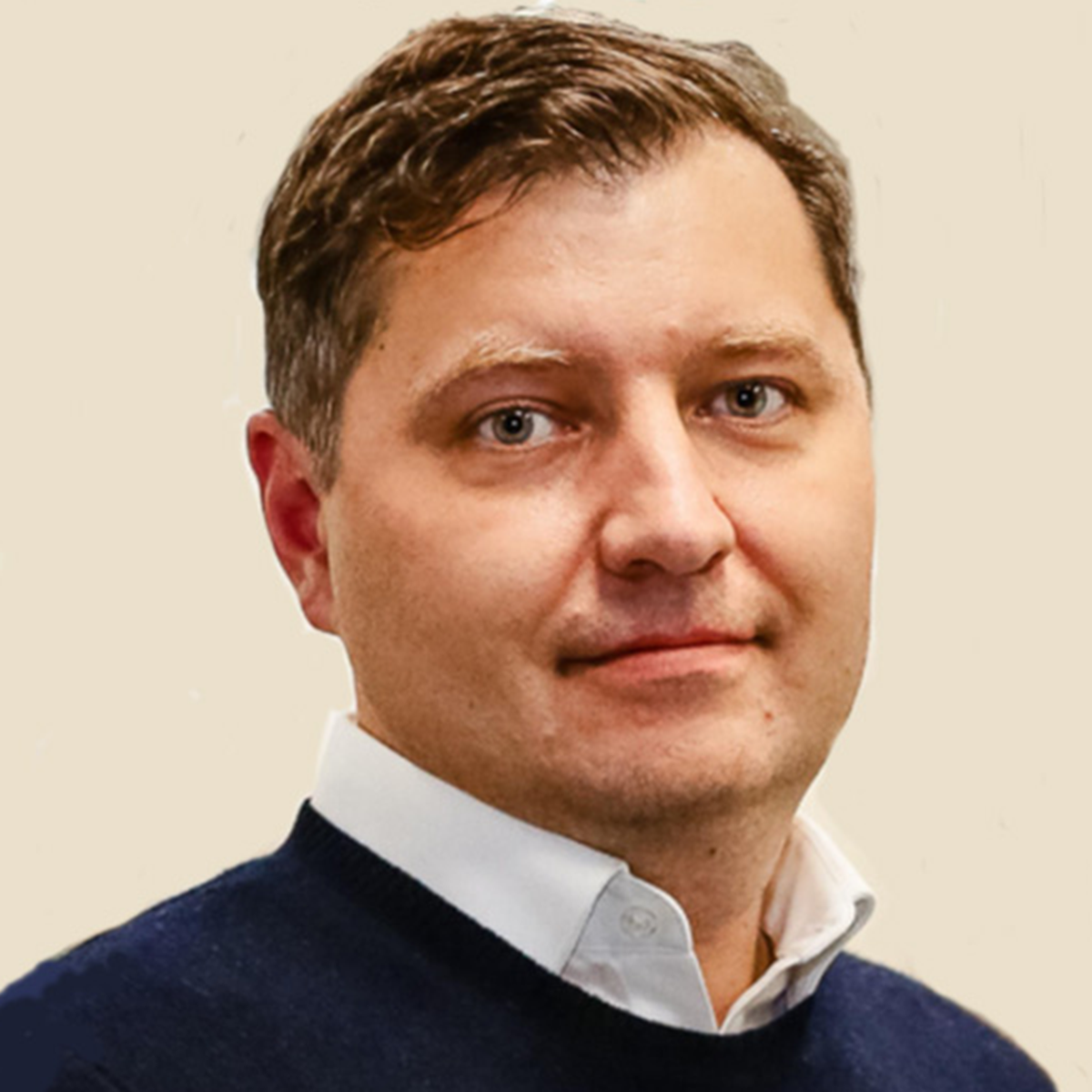
Alexey Khitrov
CEO of biometric provider ID R&D
Today, the main risk of using biometrics is not inaccuracy, but ensuring the protection of sensitive personal data. Any potential intrusion of privacy, real or perceived, risks the reputation of the companies using the technology, and has the potential to seriously damage customer trust. But banks and financial institutions base their business on trust – so how can these companies and institutions make sure they work with trustworthy providers who won’t misuse personal information and cost them their reputation?’
In 2020, the American public woke up to news about a secretive company, Clearview AI, that supplied biometric-identification systems to law enforcement. Its secret? It scraped millions of user photos from social media into its database, so they could be searched to find suspects. The problem was not the accuracy – Clearview AI has one of the most accurate facial recognition algorithms in the world – but the perceived disregard for privacy. The company was slapped with a number of lawsuits from both social networks and users, and it continues to receive huge fines from regulators tasked with protecting personal data. But it fights back, claiming that many regulators do not have jurisdiction over the data use.
Last year, the US Internal Revenue Service (IRS) started allowing citizens to log into its webpage via the customer-friendly ID.me service, which enabled users to log in with just their face instead of a more complicated vetting system. However, the IRS stopped the service almost immediately following an uproar from data protection watchdogs, who claimed the system violates citizens’ right to privacy – especially as ID.me doesn’t only match an individual’s face to a previously stored ID photo, but it does one-to-many facial recognition: comparing a facial image (and thereby adding it) to a mass database of facial images.
Again, ID.me’s algorithm is top-notch and accurate, for which the company has been repeatedly praised. The state of California, for example, claims that ID.me’s system protected USD $125-billion worth of employment fraud. Still, the uproar about the potential misuse of client data pushed the IRS to stop using ID.me for the time being.
The financial services are also not immune. Banks, for example, use AI to aid decision making when giving out loans. Some have already been accused of being biased against certain groups of customers, mostly because of the biased statistical data used for training the AI. When it comes to using biometrics for customer onboarding, banks don’t want to be facing the same problem again.
“If the banks were suddenly seen as untrustworthy, the whole system would lose its appeal as a seamless, accessible way of identification.”
Avoiding the Loss of Reputation
These examples show a clear trend: with the fast-growing use of biometrics, the main perceived risk has switched from the accuracy of the technology, to doubts over the proper use of the biometric data – which is considered sensitive personal information that requires extra protection. Just a shadow of a doubt over its proper usage can tarnish the reputation of the institution using it – e.g. a bank, a telco operator or a government agency. And this is a steep cost to an institution that is adopting the technology in order to profit from the simplification of the customer onboarding experience.
Private companies in particular want to avoid reputation loss. “Banks are seen as a source of trust, even more so than government agencies,” explains Miguel Traquina, CTO of iProov, one of the providers of biometric solutions. He points to the BankID system in Sweden as an example – which is run by the banks and used by 98% of Swedes as a source of reliable identification, thus making access to thousands of services much easier. “Through it, the organisations implicitly trust each other,” he adds. If the banks were suddenly seen as untrustworthy, the whole system would lose its appeal as a seamless, accessible way of identification. “Proper onboarding is just the tip of the iceberg when building trust, and each transaction then builds it up,” he concludes.
When There Is No Reputation to Protect
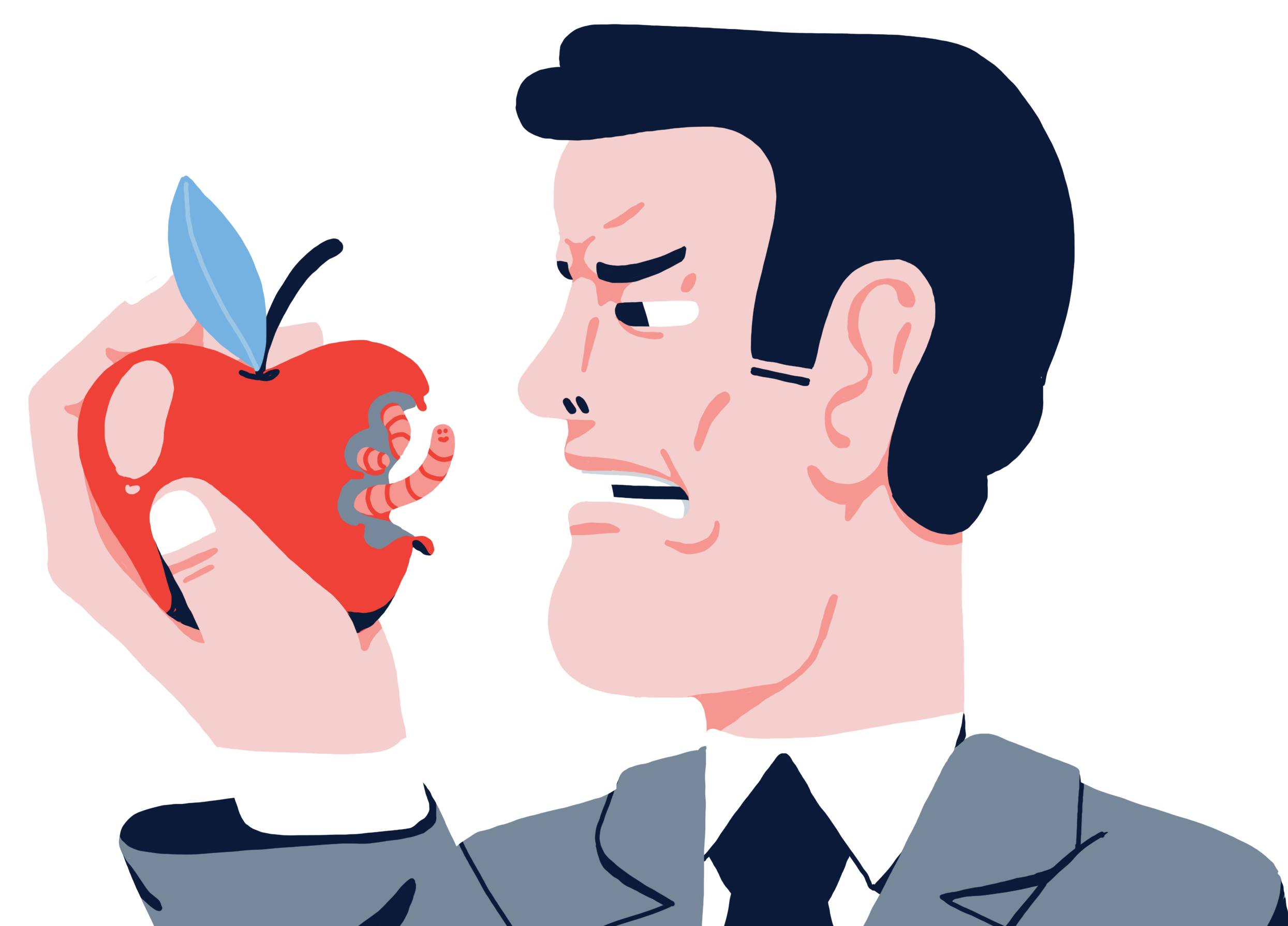
The mass quarantines during Covid-19 showed banks, as well as many other companies, that remote onboarding is a crucial technology in order to keep new customers flowing in, even if branches are forcibly closed. This sudden demand in turn created a supply of companies catering to it. In 2021 alone, these companies received fresh funding of over US $4 billion dollars from investors, showing the appetite for these solutions.
However, the number of biometric algorithm developers has not grown in stride. In fact, most of the new providers just licence existing algorithms instead of developing their own. “Many of the new providers have no reputation to protect, so they use misleading or outright false marketing claims just to sell their solutions,” says Peter Martis, head of global sales at Innovatrics. From overblown statistics to the use of open-source algorithms (which do work, but have many flaws – from lower accuracy to biases) there are many tricks that some of the newcomers can use.
“Many of the new providers have no reputation to protect, so they use misleading or outright false marketing claims just to sell their solutions.”
Benchmarks to the Rescue
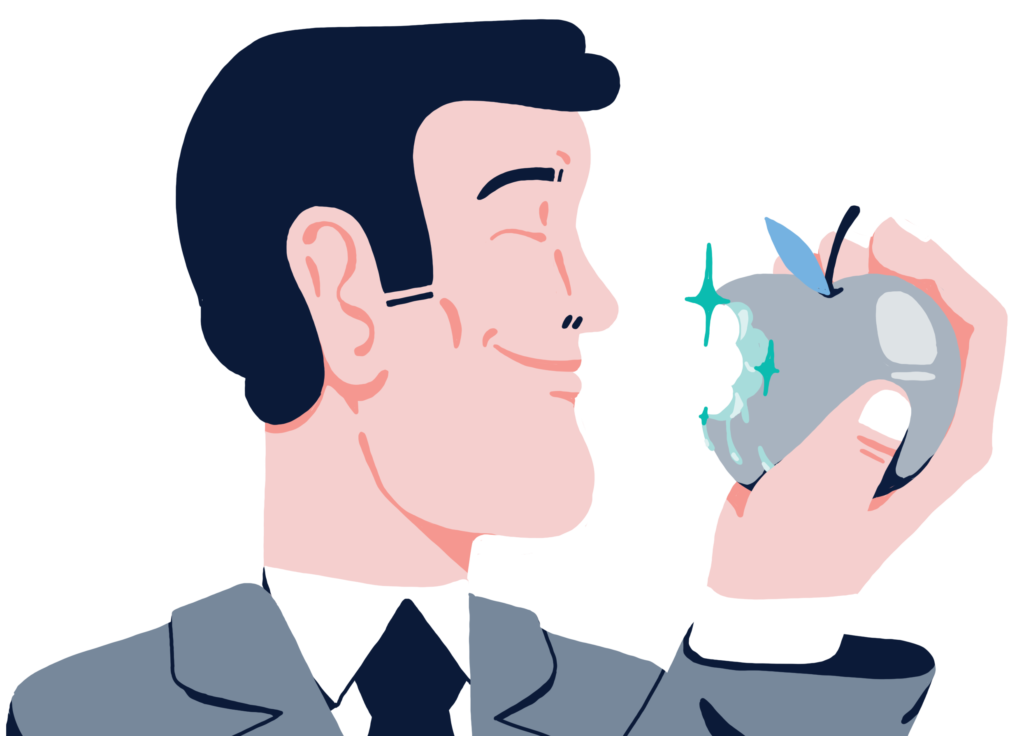
Luckily, biometrics is specific in the fact that it has a reliable, third-party means of comparing the quality of the algorithms. The US National Institute for Standards in Technology (NIST) has a number of benchmarks that concern biometric algorithms. A good rule of thumb is to always search for the algorithm of every provider who approaches with a new solution. Some of them are missing, pointing to the fact that they either use someone else’s algorithm, or their algorithm has not passed the NIST muster.
This doesn’t only concern small and upstart providers: IBM, for example, didn’t have a NIST entry before backing away from facial recognition two years ago. And Amazon, despite providing facial recognition capabilities, doesn’t have a benchmark so far, citing technical differences. All the long-time developers do provide all of their algorithms for thorough testing though. In facial recognition alone there are over 200 entries. On the other hand, iris recognition, despite being the most accurate modality, has just a handful of algorithms.
Even Bias Can Be Measured
Apart from rating the performance of fingerprint, biometric and iris recognition algorithms, NIST also does comparisons that have become increasingly important with the increased focus on private data. With the growing concern about inherent biases of the AI used in public spaces, facial recognition has come under scrutiny as well.
There are inherent biases in facial recognition that are known to the developers of these technologies – darker skin reflects less light and makes shadows less visible, which in turn causes more difficulties in detecting facial features. The second source of bias is the training of the datasets themselves: many of the freely or commercially available datasets are statistically skewed towards Caucasian faces. When neural networks are being trained, they are less exposed to darker-skinned faces and can perform poorly.
“A lot of the freely or commercially available datasets are statistically skewed towards Caucasian faces. When neural networks are being trained, they are less exposed to darker-skinned faces and can perform poorly.”
Long-term developers do know about these limitations and know how to avoid them. The main way is to provide a more varied dataset, lowering the bias overall. Larger datasets also provide an added benefit: they make the algorithm more accurate. The second way is to use technology to solve the problem. One of the larger providers, Idemia, uses synthetic faces, generated by a special algorithm. These can then fill in the gaps in datasets where certain skin types are missing or underrepresented.
Synthetic faces
Neural networks can be trained to generate lifelike faces that do not belong to a specific human. The trouble with using photos of actual people in different scenarios is usually that their consent is needed. With bias being discussed more in facial recognition, people have become more apprehensive about wanting to participate in datasets. Synthetic faces overcome this hurdle. Today, they can be used for illustration in magazines or for photo montages. One example of how powerful this generating of faces is, can be found here.
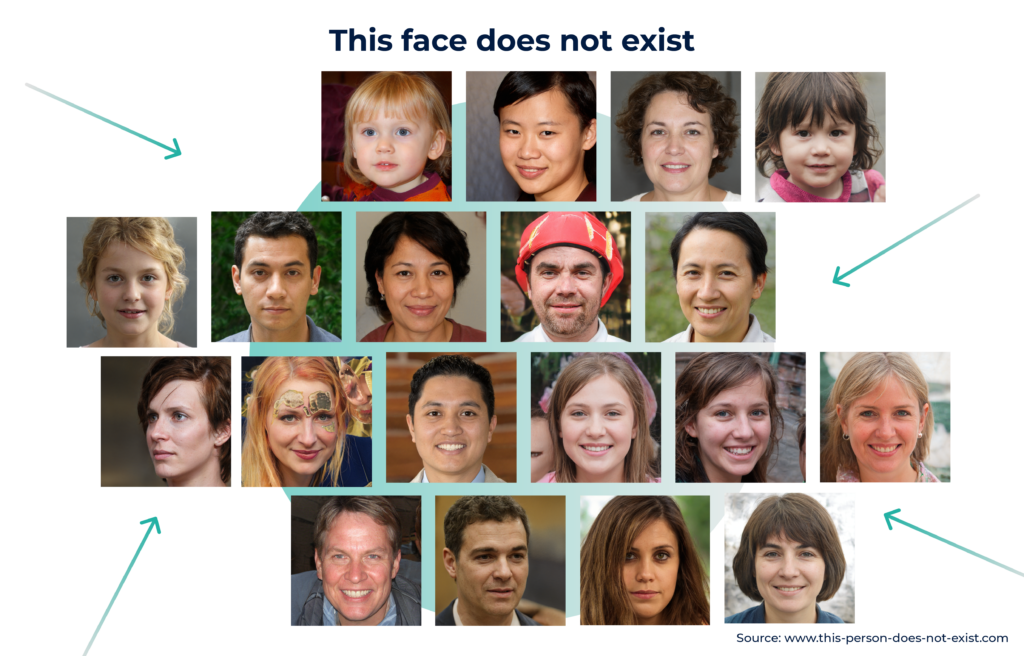
As with the accuracy measuring, NIST also measured the bias of all the algorithms in the facial recognition benchmarks. The results varied wildly, from negligible to pronounced differences in accuracy depending on skin colour. One overall rule of thumb can be drawn from the testing though: the more accurate an algorithm, the lower bias it has as well. “If a customer, say a bank or telecoms company, wants to onboard a varied, multicultural population of users, they should check out the bias comparisons of their providers. If the algorithm works poorly for a specific group of people, it would mean that the company would stand to lose a proportion of potential customers,” adds Peter Martis from Innovatrics.
“The more accurate an algorithm, the lower bias it has as well. If the algorithm works poorly for a specific group of people, it would mean that the company would stand to lose a proportion of potential customers.”
Bias is not only a problem when recognising faces, but it affects other areas as well – most importantly the liveness check. These are vital for the so-called KYC (Know Your Customer) industries, especially finance and telecoms – as they are legally obliged to make sure that their customers are who they say they are. In an online world, making sure that a person is not posing with a photo or a mask over their face is critical. Alexey Khitrov, CEO of biometric provider ID R&D, explains that for AI to be trained responsibly, there are some basic principles to be upheld. “You have to be able to identify the gaps in datasets used for machine learning and fill them up with new real or synthetic data,” he explains. The company used this approach to improve their presentation attack detection algorithm (which is a synonym for liveness check).
The biometric landscape:
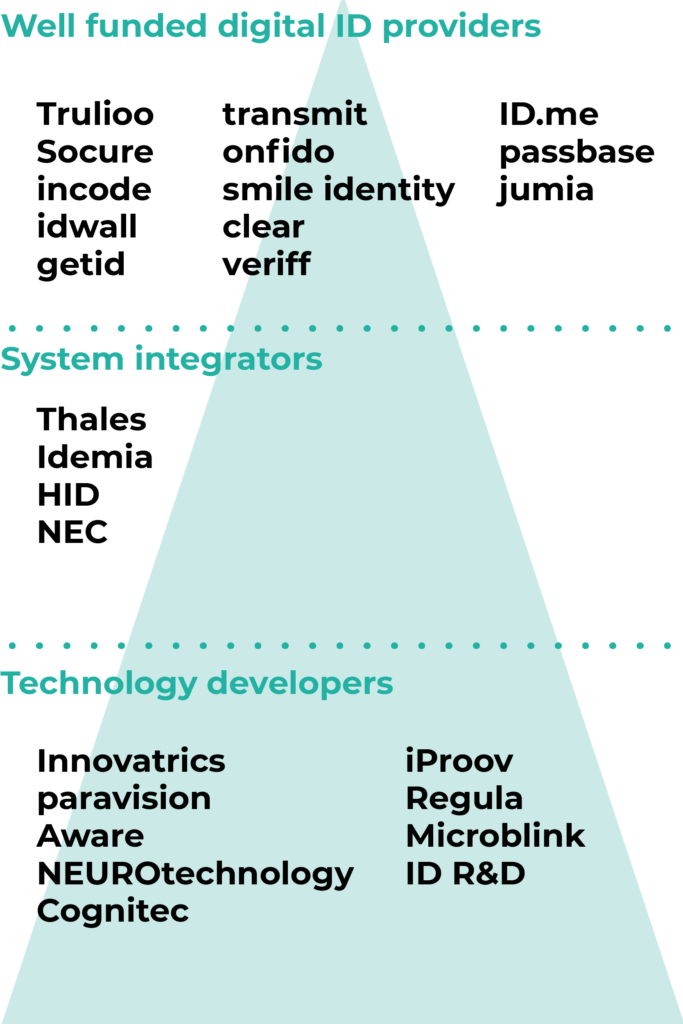
There are a handful of core technology developers that create, maintain and improve their own biometrics and other algorithms. The system integrators may have their own algorithms as well, or buy them from core tech developers. Most of the digital ID providers don’t have their own core technology, but licence the existing algorithms, thus creating a dependence on the companies below them in the pyramid.
The Data Is Out There
Although a relatively recent development, the liveness check already adheres to standardised testing. A NIST-approved company called iBeta tests liveness algorithms and provides detailed protocols on the outcome. The tests come in two levels – the first concerns only the basic attempts to fool the system with e.g. a photo cut-out of the face. Level 2 then also takes into account latex masks and more complex fraud.
To get the iBeta stamp, the liveness check must not allow any fraud at level 1 and a maximum of 1% of fraud at level 2. The iBeta protocols are a great antidote against the mentioned marketing claims: instead of trusting a startup company proclaiming a 99.98% success rate in liveness, it is easier to just check how their iBeta tests went (if there were any at all). At the time of writing this article, NIST itself has announced that it is aiming to develop a comprehensive battery of tests for liveness as well. This will put most of the necessary technology tests for digital onboarding under a single, widely trusted roof.
“Instead of trusting a startup company proclaiming a 99.98% success rate in liveness, it is easier to just check how their iBeta tests went.”
Checklist
Check the accuracy of the algorithm provider in NIST FRVT 1:1
Check the biases of the algorithms
Check the performance of liveness checks at iBeta
Test the OCR functionality on the IDs you expect future customers to use
For a more detailed checklist for procuring a digital onboarding solution, go here.
Teaching the Machine to Read
The only thing to be left out from under the NIST wings is the quality of optical character recognition (OCR). These algorithms have also undergone a revolution with their introduction to neural networks. They are used for reading IDs, and the more accurate they are, the fewer errors are introduced to the client database, avoiding problems further down the road.
As with faces, OCR has its biases as well. It reads Latin scripts exceptionally well, but the more exotic the scripts get, from Arabic to Chinese, the more difficult it is to find a proper OCR method for them. “When searching for the support of specific IDs, the only thing to recommend is good preliminary testing before deploying the solutions. Again, if customers will not be able to properly scan their IDs most of the time, they will just abandon the whole process,” Peter Martis advises.
To avoid trouble, several companies have deployed auxiliary technologies that capture the ID pictures in optimum quality to make them easier to read. This reduces the guesswork of the client while increasing the quality of the automated reading. All in all, the faster the whole process is, the more end users complete it. “Innovation is useless if it doesn’t benefit the customer in some way,” explains Miguel Traquina, CTO of iProov. Helping the end user finish the whole procedure quickly, safely and satisfactorily is the combined desire of both biometric providers and their customers.
AUTHOR: Ján Záborský
ILLUSTRATIONS: Matej Mihályi