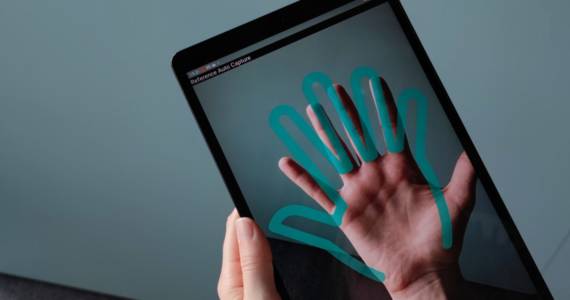
Innovatrics Enables Palm Recognition via Common Cameras, No Extra Scanner Needed
Innovatrics is the first to offer mobile palm recognition technology that requires only a mobile phone or an ...
Read moreWith proper 3D modeling, contactless fingerprints can be significantly more accurate than just comparing photos with a gallery. Here’s how we created an algorithm three times as accurate as simply taking a finger photo and comparing it to a fingerprint gallery.
One of the in-demand features in the current smartphone world is the ability to compare photographed fingerprints to the ones stored in the database. Apart from the obvious potential drawback caused by the varying photo quality, a simple comparison between a photo and a sample produces lots of errors. The reason? Traditional scanned fingerprints are pressed to the scanner bed, causing deformation of the fingerprint patterns.
Moreover, the way the ridges are seen is different: while the scanner captures their direct imprints, the photograph shows them as shadows. In effect, comparing contactless fingerprint photos and their database samples is nowhere near as accurate as comparing two scanned fingerprints. In cases where contactless fingerprints are currently being used, they usually compare photographed fingerprints to the database of photos, not scans, limiting the usefulness of this method.
“We were looking for a reliable way to compare contactless fingerprints with existing databases, as this is one of the most requested features in the fingerprint world. Instead of taking fingerprints for identification at the police station, you simply take a photograph of the fingerprints, saving a lot of time and effort,” explains Igor Janos, the Head of the Synthetic Data Team at Innovatrics, as he guides us through the steps needed to get to the final neural network.
Step 1: Cast the fingers of your colleagues
If you need to know how real fingers are deformed when being scanned, first you need fingers. Lots of fingers. Specifically, their casts in extremely fine gypsum to capture all the details. Luckily, being a biometrics company, Innovatrics has lots of volunteers at hand, willing to donate their fingers for research purposes. (No fingers were harmed during this task.) “Finding a good mix of gypsum was the first challenge – we needed one that did not contain any bubbles and showed all the features without being too brittle,” Igor Janos explains.
Step 2: Spin those fingers in a 3D scanner
In order to transfer all those detailed ridges from finger casts to the digital world, you need a 3D scanner. Luckily, the AI-powered world now offers lots of replacements for a dedicated machine. You can make a detailed video of the object you want to transfer to 3D and the online services will crunch it to produce a perfect digital replica.
Step 3: Play with fingers in Unity
Once turned into digital models, the fingers can now be played within 3D modeling programs such as Unity. The main aim? Properly model how a finger photo is transformed into a flat, scanned fingerprint. Once the connection is established, the neural network can learn how to translate a photo of a finger to a scanned fingerprint. “This was a tricky one, finding a proper material firmness to model the behavior of a live human finger,” says Janos.
Step 4: Try. Fail. Try again
As Silvester Kosmel (Igor’s colleague) adds, it took about “three months of lots of trial and error” to find a reliable approach of translating fingerprint photos to their scanned images. In the process, the digital fingers have been adorned with synthetically generated fingerprints to produce a robust dataset. ‘We have devised our own shader to be able to render the fingerprints quickly and correctly,” adds Kosmel. The resulting neural network, when it sees the finger in the camera, is now producing its scanned print in real time and can be compared to a fingerprint gallery.
Step 5: Test and measure
The last step, of course, is measuring the quality of the outcome. “The results depend heavily on the smartphone camera, but even a low-end smartphone with our neural network shows higher accuracy than a simple comparison of fingerprint photo to a scan,” says Janos.
Police fingerprint scanners have a resolution of 500 DPI or higher, making them extremely precise. Smartphone camera resolution differs by each maker. The best results have been obtained with an iPhone camera, but any photo can be translated into a 500 DPI fingerprint scan. Compared to a simple photo-to-gallery comparison, the neural network is up to three times more accurate – even with lower-end cameras.
The next step? Incorporating the neural network into mobile libraries and ABIS so our clients can benefit from the newly developed technology.